I grew up as an undergrad math (nascent computer science) major, took a detour to the school of engineering and technology, and wound-up back in the school of science, but this time with psychology as my major and a sheepskin noting a bachelor’s degree in that aforementioned science. My conversion to psychology was via a 101 course in Psychology as a Social Science. Intellectually smitten, I next took 102 Psychology as a Biological Science. You may see a theme emerging.
One of my most enjoyed courses was a 200-level methodology class. Therein we learned of the impact of one’s biases and how to guard against their intrusion, what makes for a good (and bad) study design, sampling approaches and sizes, and never proving anything but rather disproving the null hypothesis. I enjoyed this even more than methodology’s sibling, statistics, and it’s controlling for contaminating variables and sussing the probabilities of what we thought we found out to be untrue.
The moral of the scientific method’s story was that when everything is done properly, you get science. From science, you get trust. From trust, you can make judgments about proper actions to take. From making those actions, you then get the expected, predicted, recurrent result. Or so we thought.
But something’s not right….
Seventy percent of 1500 scientists surveyed in a study published in 2016 in Nature, could not reproduce the same outcome in at least one other scientist’s experiment. Furthermore, half could not even replicate their findings when running again.
What?
Perhaps one of the most recognizable researchers in a semi-new area of research called metascience (the scientific investigation of scientific investigation), Ioannidis, a Stanford statistician and professor of medicine, found that the majority of health-related studies published in the last 10 years could not be replicated and that around 17% of the studies examined were subsequently contradicted in replication studies.
This does not seem to be limited to healthcare, psychological and medical studies. Indeed, metascientific investigations have found evidence for reproducibility problems in sports science, marketing, economics, and even hydrology.
My first specialty, clinical psychology has taken this hit particularly hard. Unfortunately, other psychological disciplines have also found problems as well, including social psychology, developmental psychology, and educational research.
This just got real
So, if you cannot trust studies on pollutants, how can you craft environmental policy? If you cannot trust studies on teaching approaches, how can you better design educational curricula? If you cannot trust clinical trials on a psychotherapeutic approach or promising medication, how do you accurately instruct graduate or medical students, or properly treat patients, construct clinical guidelines, moderate doses, and protect against untoward side-effects?
If the evidence-base is off, then the treatment guidelines predicated on them will likewise be suspect, and perhaps even more concerning—iatrogenic.
So how did this happen?
Cargo Cult Science
This was the title of Richard Feynman’s commencement address to the Cal Tech class of 1974. Therein he notes the psycho-sociological phenomenon wherein during World War II, a community of Pacific Islanders misinterpreted the observational data at hand of a runway, towers, and crewmen seeming to be the causal aspects of producing the result of food staples and other beneficial cargo, floating down from the heavens under parachute canopies that were shared with them. Once the war was over and the troops departed, the indigenous people created their own runway, towers and mimicked headset equipment in order to have the same resultant cargo come raining down.
Now, we can sit back and tut-tut such a silly expectation, but Feynman points out that many of us may first fall in love with our theories and models. We may personally, professionally, and economically invest in their (and us) being right. We look for any and every particle of evidence in support of showing the world it is so, and by proxy, how smart and cool we are. In other words, we build really, really nice runways and towers.
It’s hard to admit bias. Heck, by definition, a blind spot is something unable to be seen. So isn’t it hubris to think such things don’t insinuate themselves into our scientific processes as well?
Sometimes it’s dishonesty
It’s one thing to be blinded by one’s own desire to be right about something important. It’s another to just make stuff up. Yes, folks do that, and they get published.
While I’ll not psychoanalyze theories as to why some do this, let’s look at a study. [Quick pause. I understand there is a great irony in my citing any research finding in an article on not being able to trust research findings. Quite meta, but be that as it may….] Fanelli found that in the anonymous studies she did, there was an admission rate of 1–2% for falsifying or fabricating data. Similarly, Ioannidis found few cases of misconduct in studies looking at the reproducibility of findings.
Sometimes it’s being dumb
There is a term for this—QRP, or questionable research practices. Echoing Fanelli’s and Ioannidis’ opinions, such is considered to not be too wide-spread or impactful. Phew.
Sometimes it’s p-hacking or playing in Anscombe’s Quartet
Head, Holman, Lanfear, Kahn, and Jennions define “p-hacking” or “selective reporting” in their paper as occurring “…when researchers collect or select data or statistical analyses until nonsignificant results become significant.” They found that while they believe that “…p-hacking is widespread throughout science…its effect seems to be weak relative to the real effect sizes being measured. This result suggests that p-hacking probably does not drastically alter scientific consensuses drawn from meta-analyses.”
In a reference, I have bookmarked in my web browser because I need the reminder and it’s so cool, Matejka and Fitzmaurice published a paper (and quite a fun video) that is based on Anscombe’s Quartet. “They note this ‘quartet’ is a group of four data sets, created by the statistician F.J. Anscombe in 1973, that have the same ‘summary statistics,’ or mean standard deviation, and Pearson’s correlation. Yet they each produce wildly different graphs.” For example:
The dataset called the Datasaurus, “like Anscombe’s Quartet serves as a reminder to the importance of visualizing your data, since, although the dataset produces ‘normal’ summary statistics, the resulting plot is a picture of a dinosaur. In this example (they) use the datasaurus as the initialdataset, and create other datasets with the same summary statistics.”
Schwab notes that “Matejka and Fitzmaurice made 200,000 incremental changes to the ‘Datasaurus’ data set, slightly shifting points so that the summary statistics stayed within one-hundredth of the originals. GIFs that show the slowly shifting points next to the summary statistics hammer their point home.”
Sometimes it’s the half-life of facts
As I have opined in the past, in the popular media, we all read about how this or that food or activity is alternatingly good or bad for us. In an article I wrote on How to Protect Yourself from Fad Science dealing with how to figure out what to do concerning tweaking our behavior in the context of the latest healthcare headline, I stated “…like most things, the answer is the ultimate in unsatisfying—it depends. We like to have things clear, specific and definitive. Easy to understand and readily meme-able. So, when something we like to eat that was formerly a sin is now reported to be a blessing, then we feel we have the proof for what we knew all along and we feel vindicated with the seal of science.”
But as we learned in frosh biology (or was it genetics?) “…not all treatments work the same in all people, if they did the world would not need NSAIDs in addition to aspirin. Ditto that for exercise, diet, learning, and most anything else, except for maybe math. We’re complex beings to which one size fits few. It’s more amazing to me to see something that generalizes to an N greater than one.
Ideas evolve and legitimate studies can be in conflict with other studies, without any shenanigans or ethical issues afoot.”
It reminds me of the humorous but true introduction to new medical school students…
50% of what we teach you over the next five years will be wrong, or inaccurate.
Sadly, we don’t know which 50%
Samuel Arbesman, a Harvard mathematician, coined the term “half-life of facts” in reference to the predictability of scientific papers’ findings to become obsolete. “What we think we know changes over time. Things once accepted as true are shown to be plain wrong. As most scientific theories of the past have since been disproven, it is arguable that much of today’s orthodoxy will also turn out, in due course, to be flawed.” In medical science, it can be pretty quick—by some estimates a 45-year half-life. Mathematics does a better job as most proofs stay proofs.
And sometimes it may just be Simpson’s Paradox
Simpson’s Paradox as defined in Wikipedia is a “phenomenon in probability and statistics, in which a trend appears in several different groups of data but disappears or reverses when these groups are combined.” Matejka gives the example wherein “one set of data appears to show crime increasing…yet when that data is broken down by location, there is a strong downward trend in crime in each area–another example of how data that has the same summary statistics can look vastly different when it’s graphed.” No fraud, no foul, but a good cautionary tale vis-à-vis interpretation and conclusion.
Was this self-inflicted?
So did the dynamic that spawned the “publish-or-perish” mantra/ethos/environment/ecosystem iatrogenically create legitimate scientific publishing’s doppelganger of predatory publishing in which rigor (and ethics) go out the window?
If academic promotions and status and tenure are all predicated getting in print, then it should be no surprise that non-academic, but quite nefarious “entrepreneurial” publications have resulted. It reminds me of the development of vanity presses for those with a manuscript so poor in quality that a legitimate publishing house wouldn’t give the time of day.
Predatory journals, yup, they’re a thing
I’ve written elsewhere on this “nefarious practice that has come about in the last decade known as “predatory journals” or “predatory open-access publishing.” The gist is that for a fee (and likely a steep one), someone can submit a manuscript of questionable quality and get it in print with little or no serious editorial peer-review. New academics or those gunning for tenure or promotion need to bolster their CVs with publications, as publish-or-perish continues to be alive and well in ivory towers and ivy halls, and this is a bad shortcut. Newer faculty and those from the developing world may not be aware of how to differentiate a legitimate journal from a predatory one. While this is exploitative to the submitting author, it also prevents the educational aspect of what a rejection from peer-review can provide to improve a methodological approach, hypothesis construction and testing, or writing ability. Of greater concern is that it also has the potential to do harm if a poorly vetted medical approach or diet, for example, becomes adopted.”
Tips to Tell the Difference
I’ve written previously on this and here are some tips that may be of help:
Is the journal for real and high quality?
First off, is the journal legitimate? You can look here for suspicious journals. If the journal is fine, then see what its Impact Factor is. Impact Factor is “a measure of the frequency with which the average article in a journal has been cited in a particular year. It is used to measure the importance or rank of a journal by calculating the times its articles are cited” as my University notes. There are additional tools like Eigenfactor, SCImago Journal Rank (SJR), and Scopus. All of these and more are detailed here.
Google Scholar recently made publicly available “Scholar Metrics (which) provide an easy way for authors to quickly gauge the visibility and influence of recent articles in scholarly publications… This release covers articles published in 2014–2018 and includes citations from all articles that were indexed in Google Scholar as of July 2019 (and) include journals from websites that follow (their) inclusion guidelines and selected conferences in Engineering & Computer Science. Publications with fewer than 100 articles in 2014-2018 or publications that received no citations over these years are not included.
Is the author for real?
Healthcare practitioners have to have licenses in order to practice, and the majority of healthcare researchers and scientists are part of an established university, research, or corporate entity. Anyone that is a licensed healthcare provider or proper academic researcher will thus be super easy to find out about via Google or Google Scholar. In most things, but especially in healthcare, education, training, qualifications/licensure, and experience really, really do matter.
In fact, speaking of Google Scholar, they have some spiffy metrics vis-à-vis one’s scholarly productivity and impact. Here’s a sampler of mine noting h index and i10 index scores:
From the other side of the desk
Over 25 years ago I wrote the book From the Other Side of the Couch about what is it like to be a psychologist or a psychiatrist, different kinds of practices and training, the effect that clients had on them, how they handled ethical questions, and so forth.
I have now sat on both sides of the “desk” when it comes to publishing. That is, as a hopeful scientist, submitting my manuscript to microscopic scrutiny and in later years as a peer reviewer on scientific journal editorial boards and book editor for the likes of Wiley, Praeger, Greenwood, Charles C. Thomas, and other long-standing and respected publishing houses.
And I am here to tell you, quality varies in submissions. Oftentimes, a lot. But, a model I have seen work very well, and that I first experienced via my dissertation process, was that of having a Dissertation (or Review) Committee made up of diverse experts. For example, I had one member whose expertise was statistics and methodology design (vetting my science), another evaluated my theory and thinking, and the third critiqued and helped me with my writing and communication. Once they were all happy with it, I presented to a group naive to my work for fresh eyes and ideas for a final evaluation and recommendations. In book projects, I have sent out chapters that I did not have the proper skill-set to evaluate, out to a blind content expert for peer-review. In journals, I could also make a similar request on a submission that may have an area I was not proper to comment on or evaluate. Every paper I ever reviewed was likewise reviewed by another colleague with complementary abilities and expertise, and our independent, blind reviews went up to the food chain of other editors before a final yea-or-nay by the Editor in Chief.
The File Drawer Problem
The phenomenon is known as “The File Drawer Problem” or “Publication Bias.” This means that a researcher whose study “fails to reject the null hypothesis (i.e., that do not produce a statistically significant result) (is) less likely to be published than those that do produce a statistically significant result.” Thus, the study is relegated to the file drawer, rather than the printing press.
Furthermore, it’s hard to calculate how many studies are never even submitted due to the presumption of rejection for not gaining statistical significance. I call this the “why even bother dilemma.” It takes a great deal of time, effort, and cost to do research—the flops as well as the successes. If one presumes a likely rejection by virtue of having insignificant findings, then why in the world would you “waste” more time and money to write it up? Instead, why not spend that time and effort on a more promising dataset?
Unintended Tower of Babble?
Earlier in my career, I focused on clinical outcome studies in behavioral health and published a fair amount. To me, such research serves as the foundation from which to understand what seems to work well and then build out treatment guidelines, and thus support the ability to inform evidence-based practice.
But in my later work in general medicine, orthopedic medicine, arthroscopic surgery, pain medicine, oncology nursing, and others, I found there was no lingua franca for reading a journal in any of the specialties and understanding their context for an evidence base. For example, an evidence rating of “Grade B” from the Agency for Healthcare Research and Quality would equate to a “Level III” in an arthroscopic surgery journal, or be termed “Weak Recommendation” in a general medicine journal. This made me crazy, so I needed to develop a translational method and developed what I call the Rosetta Stone for Comparing Levels or Grades of Evidence in Various Healthcare Professions. It’s freely available here.
Well, that was disappointing
Preregistration or “publicly” registering a plan of research is one way in which psychology scientists and others are working towards a solution to the problem of reproducibility. Basically, a “…registered report format requires authors to submit a description of the study methods and analyses prior to data collection. Once the method and analysis plan is vetted through peer-review, the publication of the findings is provisionally guaranteed, based on whether the authors follow the proposed protocol. One goal of registered reports is to circumvent the publication bias toward significant findings that can lead to the implementation of questionable research practices and to encourage publication of studies with rigorous methods.”
But, early returns, at least for psychology, are a bit disappointing. In an article by David Adam, entitled “A solution to psychology’s reproducibility problem just failed its first test” he described the experience of Aline Claesen, a psychologist at the Catholic University of Leuven (Belgium), who with her team, looked into 27 preregistration plans for studies to be considered by the journal Psychological Science. Claesen’s findings? Every single one of the 27 “…researchers deviated from their plan—and in every paper but one, they did not fully disclose these deviations.”
To be fair, this is a nascent approach that may have some kinks to work out, and it will take time to adopt this new approach to become a norm and more universal to more and more journals. Dan Simons, a psychologist and faculty at University of Illinois, Champaign-Urbana noted, “My guess is that most [authors] were well-intentioned and just didn’t know how to do it very well.”
New Rules for the Road
Diener and Biswas-Diener suggest Dissemination of Replication Attempts, for example:
“Center for Open Science: Psychologist Brian Nosek, a champion of replication in psychology, has created the Open Science Framework, where replications can be reported.
“Association of Psychological Science: Has registered replications of studies, with the overall results published in Perspectives on Psychological Science.
“Plos One: Public Library of Science—publishes a broad range of articles, including failed replications and there are occasional summaries of replication attempts in specific areas.
“The Replication Index: Created in 2014 by Ulrich Schimmack, the so-called “R Index” is a statistical tool for estimating the replicability of studies, of journals, and even of specific researchers. Schimmack describes it as a “doping test.”
“Open Science Framework: an open-source software project that facilitates open collaboration in science research.
“Psych File Drawer: Created to address the file drawer problem and allows users to upload results of serious replication attempts in all research areas of psychology. Archives attempted replications of specific studies and whether replication was achieved.
They go on to make the point that “The fact that replications, including failed replication attempts, now have outlets where they can be communicated to other researchers is a very encouraging development, and should strengthen the science considerably. One problem for many decades has been the near-impossibility of publishing replication attempts, regardless of whether they’ve been positive or negative.”
Open Science has Six Principles as guides for better scientific inquiry:
“Open Methodology: document the application of methods as well as the entire process behind as far as practicable and relevant documentation.
“Open Source: Use open source technology (software and hardware) and open your own technologies.
“Open Data: Make data freely available.
“Open Access: Publish in an open manner and make it accessible to everyone (Budapest Initiative).
“Open Peer Review: Transparent and traceable quality assurance through open peer review.
“Open Educational Resources: Use Free and Open Materials for Education and University Teaching
Ioannidis offers some helpful tips in the form of Corollaries: to keep in mind when conducting, reviewing or reading studies:
“Corollary 1: The smaller the studies conducted in a scientific field, the less likely the research findings are to be true.
“Corollary 2: The smaller the effect sizes in a scientific field, the less likely the research findings are to be true.
“Corollary 3: The greater the number and the lesser the selection of tested relationships in a scientific field, the less likely the research findings are to be true.
“Corollary 4: The greater the flexibility in designs, definitions, outcomes, and analytical modes in a scientific field, the less likely the research findings are to be true.
“Corollary 5: The greater the financial and other interests and prejudices in a scientific field, the less likely the research findings are to be true.
“Corollary 6: The hotter a scientific field (with more scientific teams involved), the less likely the research findings are to be true.”
He also notes that small sample sizes can result in heightened effects using significance thresholds. So, as with most things, bigger is better! When replications studies are done, they should use sample sizes in excess of those in the initial investigation (duh).
Sanjay Basu, a faculty member of both Harvard Medical School and the Imperial College London, recommends three approaches:
“Democratize data: While individual health data are and should be private, datasets can, with appropriate consent, be de-identified and shared while ensuring appropriate informed consent and protecting individual privacy. The demand from participants in clinical research studies to make their data available in this way has generated surprising revelations about the results of major drug trials and increased the capacity to make better decisions about health. Data sharing, code sharing, and replication repositories are typically free to use.
“Embrace the null. Null results are much more likely to be true—and are more common—than ‘significant’ results. The excessive focus on publishing positive findings is at odds with the reality of health: that most things we do to improve our health probably don’t work and that it’s useful to know when they don’t. Researchers should focus on how confident they are about their results rather than on whether their results should simply be labeled ‘significant’ or not.
“Be patient. The 19th-century physician William Osler once said, “The young physician starts life with 20 drugs for each disease, and the old physician ends life with one drug for 20 diseases.” New revelations take time to replicate, and new interventions—particularly new drugs—have safety issues that may become apparent only years after they come on the market. Older therapies may be less effective but may also be most reliably understood. If we demand that new therapies stand the test of time, we offer ourselves the opportunity to be safer as we balance innovation with healthy skepticism.”
p-hiking
David Colquhoun makes the point that using the use of a p-value of 0.05 (the universally accepted lowest level of presuming you’re onto something), means that 30% of the time, you are indeed incorrect. He recommends to “…insist on p≤0.001. And never use the word ‘significant’.”
Can Reverend Bayes help us out? Probably.
Some folks have recommended that we shift from the orthodoxy of p-values altogether and instead use Bayesian methods. Oh, if it were only that easy. Our pal, Colquhoun has pointed out that while he fosters the idea that the term “significance” no longer be used, while still keeping p-value calculations and specifying confidence intervals, the addition of the risk of a false positive should be added. Matthews suggests that one way around the prior probability problem is to use what’s known as the reverse Bayesian approach. “The aim now is to extend the results to a range of p-values, and to present programs (in R), and a web calculator, for calculation of false-positive risks, rather than finding them by simulation.”
Maybe the Reproducibility Problem is really a Falsification Void
Karl Popper is known for his perspective on falsification is the sine qua non of scientific understanding rather than reproducibility. That is the belief that we can never really “prove” anything. It reminds me of Nassim Nicholas Taleb’s point with black swans—they do not exist until we see that they do.
Or, maybe we just need to circle back to our methods…
Feynman elegantly proffered having scientific integrity, and “if you’re doing an experiment, you should report everything you think might make it invalid…the idea is to try to give all of the information to help others judge the value of your contribution; not just the information that leads to judgment in one particular direction or another.” He also said
Don’t fool yourself, and you’re the easiest one to fool.
Point taken, Richard, point taken.
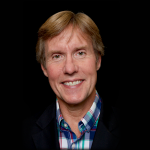
Dr. Chris E. Stout
Dr. Chris Stout is Vice President of Clinical Research and Data Analytics at ATI, the nation’s largest orthopedic rehabilitation and sports medicine corporation, with over 800 clinics and 23,000 patients seen daily. He has an extensive background in technology ventures with clinical overlap. He’s held academic appointments at Northwestern University’s Feinberg School of Medicine, the University of Illinois at Chicago’s College of Medicine, and Rush University. Dr. Stout was educated at Purdue University, The University of Chicago’s Booth Graduate School of Business, and Forest Institute, where he earned a doctorate in clinical psychology. He was a Post-doctoral Fellow in neurodevelopmental behavioral pediatrics at Harvard Medical School. He has received four additional doctorates (honoris causa) including a Doctorate in Technology. He’s a bestselling author and also published software developer. He’s lectured worldwide and was an invited faculty by the World Economic Forum to Davos. He is a popular LinkedIn Influencer and writes on global health, medicine, and humanitarian activism, with over 440,000 followers. He also writes for Geek.ly on technology, big data and predictive analytics in healthcare. Dr. Stout hosts a popular podcast that covers various topics including medical innovations from Blockchain to machine learning.